The problem DeepScreen solves
Speech activity and robocall detection using state of the art supervised learning.
Problem Statement :- On a daily basis, the Dial 112 System receives a large number of calls, with over 95 percent of them being blank, system-generated, or spoofing calls. The solution should prevent these non-productive calls on IVRS after identifying them.
I am using a technique called Voice Activity Detection to screen the call for human voice and filter the system generated calls or calls made by mistake by user applications like emergency dialers.
We can screen every call for the first 5 seconds to detect if the call is genuine or not and then make a decision to pass it to the police operator. I've also incorporated a feature to extract out exact timestamps in the audio where speech is detected. Try it out here.
VADs are already widely used in call-center industry to increase agent productivity . Dialers can discern whether a human or a computer answered the call by accurately setting VAD settings, and if it was a person, transfer the call to an available agent.
I expanded an existing(silero) state of the art VAD model to better detect Indian speech using a dataset generously provided by IEEE dataport.
I cleaned, standardised and organised the data (uploaded here) and used pytorch and torch-audio to retrain the exiting silero jit model. (took some help from the silero author to better understand the original dataset it was trained on).
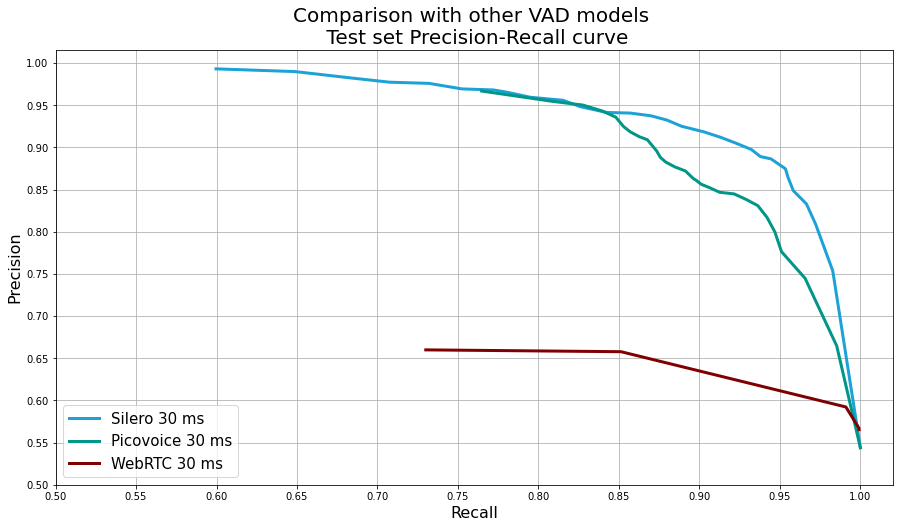
Challenges I ran into
Challenges I ran into ->
- Deploying the machine learning model on the cloud : ML model are notoriously difficult to deploy for production use. I settled with using torchscript to get a JIT model and used flask with gunicorn and ngnix to serve the webapp and secured it using ssl to make transfer of audio over network secure.
- Extending a pre-existing model with limited information. I contacted the silero's authors for help with extending the model for hindi dataset. He helped me understand the dataset, on which the original model was trained on.
- Scraping hindi t2s data and white/envoronmental noise data. Used bs4 and selenium for this job. Still need more robocaller data.
Current shortfalls ->
- The system(mic arrays) has to calibrated for every device we run this model on. This is a non issue in a standardised call centre setting but becomes and issue if we want to distribute this app to different devices. This the reason that the results of the demo website may not be accurate for your system.
- The robocaller dataset I generated/scraped together to predict machine made calls was using popular online hindi text to speech websites like this and this. I exhausted the free plan quickly to get enough data to make the models more accurate. So current accuracy for robocall detection stands at around 56% (not suitable for deployment {YET}).
- Current back-end is written in python which is great for quick prototyping but I doubt it can handle high traffic scenarios in 112 call centres. I am writing a robust audio server in golang with better concurrency support to receive and process audio data to feed it to the machine learning model.